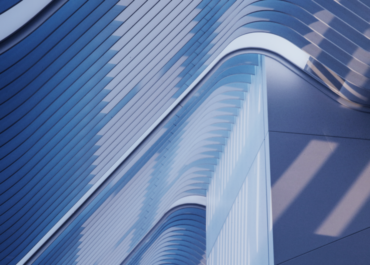
Symphony’s 2024 in review: solving for market challenges and achieving steady growth
In 2024, Symphony celebrated 10 years of supporting financial services firms drive innovation, efficiency and security as it evolved into a communications and markets technology firm. With interconnected platforms for messaging, voice, directory and analytics, and a growing community of 1,300+ institutions and 600,000+ users around the world, Symphony has established itself as the most valued financial services community.