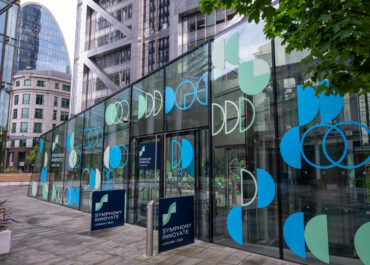
Symphony Innovate 2025 Recap | Video Highlights
Every year, Symphony Innovate brings together industry leaders to share insights, showcase live demos of products, integrations and workflow automations, as well as provide case studies on how technological advancements have transformed the community.
Innovate New York 2024 featured live demos and new innovations in action from Symphony, Citi, DTCC, Eidosmedia, HUB, LoanBook, RBC & MDX Technologies, Taskize, TP ICAP & ipushpull, UBS, Wells Fargo, 28Stone and more.